In a recent podcast from Mayo Clinic Cardiovascular services the focus was on Artificial Intelligence and especially the challenges in AI based ECG interpretation.
In this podcast (click on the link for the full podcast) Dr. Anthony Kashou MD talks with Doctors Gari Clifford and Reza Sameni. Both are AI and ECG experts and work at the Emori University (Atlanta, Georgia USA). In their discussion major challenges for making the use of AI effective in ECG interpretation are explored.
A most interesting topic since the medtech world is buzzing with AI and cardiology is no exception.
In this case the focus is not on applying AI in doctor patient communication (as the current focus regarding programs such as ChatGTP are about) but on the more deep tech quality of accurate ECG signal interpretation.
And this accuracy is still a major problem. In earlier blogs we discussed meta studies which demonstrate an average accuracy in ECG interpretation of 54%-56% among physicians.
In short the challenges discussed in the podcast are listed below and we have added our own experience and insights to this list. This makes the list of challenges even longer:
- The availability of data which can be used for building, training and testing AI based ECG interpretation solutions. The guests of Kashou mention there are large collections of data around the world that are just sitting in silos and not being shared. And for training effective AI models you do need millions of ECG data sets.
- Second these data silos are often only representative of the patient population from where they were collected. Which means there’s still very few complex large databases out there that really reflect a full spectrum of real world data. And the experts think this has really inhibited the innovation of ECG analysis.
- Another big challenge is the problem the labels of each ECG data set lack information and the data are often noisy. Many ECGs are either only machine read so they’re only as good as the algorithm used to interpret them. And it’s a known fact the traditional embedded algorithms do often not deliver an adequate result which is often below the accuracy of the physicians who work with these outputs. And so if you train a model, it’s just going to inherit the biases and inaccuracies of the original algorithm.
As for the labels delivered by human experts the challenge is these numbers are quiet low in volume and one expert ECG reader may come up with another finding they another physician. This also introduces a lot of ‘noise’ in the data. - Another challenge is the possibility of bias in the data. If a patient population isn’t well represented in the data, then you can’t learn the issues that are unique to that population.
There may be population related differences in each data set which may relate to the geographical area where they data has been collected, or to the specialism of the hospital ore team who are focused on specific cardiac disorders or other reasons. You need to verify the impact of the diversity of data before you can be sure the AI model is effective. Or to know in which cases and context the AI model delivers adequate output. - Another challenge according to the experts is the way the training of the algorithms is not aligned with the outcomes of importance that people use to assess the performance of the algorithms. These AI algorithm deliver output which are more or less a black box results which cannot be traced back to the original ECG output. And this makes it difficult for physicians to evaluate the resulting message and this means the effectiveness is based on trusting the algorithms.
- Also the experts in the podcase mention the compartmentalization of data. This makes it for instance almost impossible to link the ECG data to the results of Echocardiography (Ultrasound) or CT / MRI imaging. And with this compartmentalization of the data it is a true challenge to develop AI models which do deliver this integration.
The underlying issue is the need to add additional features to the ECG data to improve the ECG interpretation. And this is route we notice many AI attempts take to increase the quality of the model. - Finally another technical reason regarding the data quality is brought forward. There’s a great difference in types of electrodes and the level of conduction achieved during ECG recordings. Again this influences the quality of the data and the ECG data sets do not contain information regarding the quality of the electrode conduction per recording
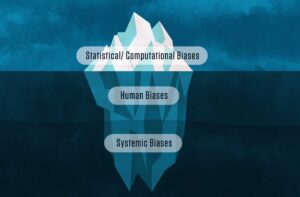
From our perspective we notice there’s no mentioning of the electrode positioning during the recording. This is another major factor influencing the morphology of the ECG waveforms. Studies have shown on average one or more electrodes are not positioned at the recommended places in 50% of the cases for Males and even 80% for Females. Again in ECG data sets the information is not available regarding the electrode positions used during the recording.
Solutions to the challenges in AI based ECG interpretation
All the AI related challenges are known in the community looking for improving ECG interpretation.
And when we started to develop CineECG we decided to evade these challenges as much as possible.
We decided not to go the AI route but to move established technologies such as Vectorcardiography and inverse cardiac modelling to next levels. This enables us to build and test our solutions with smaller volumes of ECG data sets (still >30.000) but these data sets are classified by human experts. It enables us to verify the impact of population characteristics, to handle bias in the data, etc. And through our modelling technology we can link to other datasets from Echo, MRI or CT.
And by relating the ECG data to the heart anatomy we do add a new list of features to the algorithms which are effective in increasing the much needed accuracy in ECG interpretation.
Differences between males and females are taking into account in our analysis including the variances in electrode positions.
For physicians CineECG does not deliver black box answers. You can still related the analysis results to the standard ECG output and understand what’s happening inside the heart.
As we are moving forward to our market launch we will use this blog to provide more details on CineECG in the near future. And show how we have found solutions for the challenges in AI based ECG interpretation.